图书介绍
统计和计算逆问题 英文版PDF|Epub|txt|kindle电子书版本网盘下载
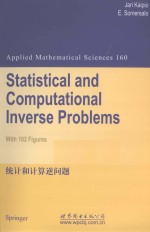
- (芬)芬兰著 著
- 出版社: 北京:世界图书北京出版公司
- ISBN:9787510086311
- 出版时间:2015
- 标注页数:339页
- 文件大小:48MB
- 文件页数:354页
- 主题词:逆问题-英文
PDF下载
下载说明
统计和计算逆问题 英文版PDF格式电子书版下载
下载的文件为RAR压缩包。需要使用解压软件进行解压得到PDF格式图书。建议使用BT下载工具Free Download Manager进行下载,简称FDM(免费,没有广告,支持多平台)。本站资源全部打包为BT种子。所以需要使用专业的BT下载软件进行下载。如BitComet qBittorrent uTorrent等BT下载工具。迅雷目前由于本站不是热门资源。不推荐使用!后期资源热门了。安装了迅雷也可以迅雷进行下载!
(文件页数 要大于 标注页数,上中下等多册电子书除外)
注意:本站所有压缩包均有解压码: 点击下载压缩包解压工具
图书目录
1 Inverse Problems and Interpretation of Measurements1
1.1 Introductory Examples3
1.2 Inverse Crimes5
2 Classical Regularization Methods7
2.1 Introduction:Fredholm Equation7
2.2 Truncated Singular Value Decomposition10
2.3 Tikhonov Regularization16
2.3.1 Generalizations of the Tikhonov Regularization24
2.4 Regularization by Truncated Iterative Methods27
2.4.1 Landweber-Fridman Iteration27
2.4.2 Kaczmarz Iteration and ART31
2.4.3 Krylov Subspace Methods39
2.5 Notes and Comments46
3 Statistical Inversion Theory49
3.1 Inverse Problems and Bayes'Formula50
3.1.1 Estimators52
3.2 Construction of the Likelihood Function55
3.2.1 Additive Noise56
3.2.2 Other Explicit Noise Models58
3.2.3 Counting Process Data60
3.3 Prior Models62
3.3.1 Gaussian Priors62
3.3.2 Impulse Prior Densities62
3.3.3 Discontinuities65
3.3.4 Markov Random Fields66
3.3.5 Sample-based Densities70
3.4 Gaussian Densities72
3.4.1 Gaussian Smoothness Priors79
3.5 Interpreting the Posterior Distribution90
3.6 Markov Chain Monte Carlo Methods91
3.6.1 The Basic Idea91
3.6.2 Metropolis-Hastings Construction of the Kernel94
3.6.3 Gibbs Sampler98
3.6.4 Convergence106
3.7 Hierarcical Models108
3.8 Notes and Comments112
4 Nonstationary Inverse Problems115
4.1 Bayesian Filtering115
4.1.1 A Nonstationary Inverse Problem116
4.1.2 Evolution and Observation Models118
4.2 Kalman Filters123
4.2.1 Linear Gaussian Problems123
4.2.2 Extended Kalman Filters126
4.3 Particle Filters129
4.4 Spatial Priors133
4.5 Fixed-lag and Fixed-interval Smoothing138
4.6 Higher-order Markov Models140
4.7 Notes and Comments143
5 Classical Methods Revisited145
5.1 Estimation Theory146
5.1.1 Maximum Likelihood Estimation146
5.1.2 Estimators Induced by Bayes Costs147
5.1.3 Estimation Error with Affine Estimators149
5.2 Test Cases150
5.2.1 Prior Distributions150
5.2.2 Observation Operators152
5.2.3 The Additive Noise Models155
5.2.4 Test Problems157
5.3 Sample-Based Error Analysis158
5.4 Truncated Singular Value Decomposition159
5.5 Conjugate Gradient Iteration162
5.6 Tikhonov Regularization164
5.6.1 Prior Structure and Regularization Level166
5.6.2 Misspecification of the Gaussian Observation Error Model170
5.6.3 Additive Cauchy Errors173
5.7 Discretization and Prior Models175
5.8 Statistical Model Reduction,Approximation Errors and Inverse Crimes181
5.8.1 An Example:Full Angle Tomography and CGNE184
5.9 Notes and Comments186
6 Model Problems189
6.1 X-ray Tomography189
6.1.1 Radon Transform190
6.1.2 Discrete Model192
6.2 Inverse Source Problems194
6.2.1 Quasi-static Maxwell's Equations194
6.2.2 Electric Inverse Source Problems197
6.2.3 Magnetic Inverse Source Problems198
6.3 Impedance Tomography202
6.4 Optical Tomography208
6.4.1 The Radiation Transfer Equation208
6.4.2 Diffusion Approximation211
6.4.3 Time-harmonic Measurement219
6.5 Notes and Comments219
7 Case Studies223
7.1 Image Deblurring and Recovery of Anomalies223
7.1.1 The Model Problem223
7.1.2 Reduced and Approximation Error Models225
7.1.3 Sampling the Posterior Distribution229
7.1.4 Effects of Modelling Errors234
7.2 Limited Angle Tomography:Dental X-ray Imaging236
7.2.1 The Layer Estimation239
7.2.2 MAP Estimates240
7.2.3 Sampling:Gibbs Sampler241
7.3 Biomagnetic Inverse Problem:Source Localization242
7.3.1 Reconstruction with Gaussian White Noise Prior Model243
7.3.2 Reconstruction of Dipole Strengths with the e1-prior Model245
7.4 Dynamic MEG by Bayes Filtering249
7.4.1 A Single Dipole Model250
7.4.2 More Realistic Geometry253
7.4.3 Multiple Dipole Models254
7.5 Electrical Impedance Tomography:Optimal Current Patterns260
7.5.1 A Posteriori Synthesized Current Patterns260
7.5.2 Optimization Criterion262
7.5.3 Numerical Examples265
7.6 Electrical Impedance Tomography:Handling Approximation Errors269
7.6.1 Meshes and Projectors270
7.6.2 The Prior Distribution and the Prior Model272
7.6.3 The Enhanced Error Model273
7.6.4 The MAP Estimates275
7.7 Electrical Impedance Process Tomography278
7.7.1 The Evolution Model280
7.7.2 The Observation Model and the Computational Scheme283
7.7.3 The Fixed-lag State Estimate285
7.7.4 Estimation of the Flow Profile286
7.8 Optical Tomography in Anisotropic Media291
7.8.1 The Anisotropy Model292
7.8.2 Linearized Model296
7.9 Optical Tomography:Boundary Recovery299
7.9.1 The General Elliptic Case300
7.9.2 Application to Optical Diffusion Tomography303
7.10 Notes and Comments305
A Appendix:Linear Algebra and Functional Analysis311
A.1 Linear Algebra311
A.2 Functional Analysis314
A.3 Sobolev Spaces316
B Appendix 2:Basics on Probability319
B.1 Basic Concepts319
B.2 Conditional Probabilities323
References329
Index337