图书介绍
管理科学导论 英文版·第8版PDF|Epub|txt|kindle电子书版本网盘下载
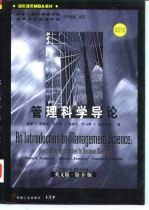
- (美)戴维 R.安德森(David R.Anderson)等著 著
- 出版社: 北京:机械工业出版社
- ISBN:7111065557
- 出版时间:1998
- 标注页数:821页
- 文件大小:76MB
- 文件页数:846页
- 主题词:
PDF下载
下载说明
管理科学导论 英文版·第8版PDF格式电子书版下载
下载的文件为RAR压缩包。需要使用解压软件进行解压得到PDF格式图书。建议使用BT下载工具Free Download Manager进行下载,简称FDM(免费,没有广告,支持多平台)。本站资源全部打包为BT种子。所以需要使用专业的BT下载软件进行下载。如BitComet qBittorrent uTorrent等BT下载工具。迅雷目前由于本站不是热门资源。不推荐使用!后期资源热门了。安装了迅雷也可以迅雷进行下载!
(文件页数 要大于 标注页数,上中下等多册电子书除外)
注意:本站所有压缩包均有解压码: 点击下载压缩包解压工具
图书目录
ContentsCHAPTER ONE Introduction1
F Answers to Even-Numbered Problems F-1
E References and Bibliography E-1
D Matnx Notation and Operations D-1
C Values of e-λC-1
B Random Digits B-1
Appendixes A-1
G Solutions to Self-Test Problems G-1
A Areas for the Standard Normal Distribution A-2
1.1 Problem Sotving and Decision Making2
1.2 Quantitative Analvsis and Decision Making3
1.3 Guantitative Analysis5
Model Development6
Data Preparation9
Model Solution10
Report Generation11
A Note Regarding Implementation11
Revenue and Volume Models12
Cost and Volume Models12
1.4 Models of Cost,Revenue,and Profit12
Profit and Volume Models13
Break-Even Analysis13
1.5 Management Science in Practice14
Management Science Techniques14
Methods Used Most Frequently15
Glossary17
Summary17
Problems18
Appendix 1.1 Spreadsheets for Management Science21
Appendix 1.2 The Management Scientist Software Package22
Management Science in Practice:Mead Corporation25
CHAPTER TWO Linear Programming:The Graphical Method27
2.1 A Simple Maximization Problem28
The Objective Function29
The Constraints30
Mathematical Statement of the Par,Inc.,Problem31
2.2 Graphical Solution32
A Note on Graphing Lines41
Summary of the Graphical Solution Procedure for Maximization Problems43
Slack Variables43
2.3 Extreme Points and the Optimal Solution45
2.4 A Simple Minimization Problem47
Surplus Variables50
Summary of the Graphical Solution Procedure for Minimization Problems50
Alternative Optimal Solutions52
2.5 Special Cases52
Infeasibility53
Unbounded54
2.6 Introduction to Sensitivity Analysis56
Obiective Function Coefficients57
2.7 Graphical Sensitivity Analysis57
Right-Hand Sides62
Glossary64
Summary64
Problems65
Case Problem:Production Strategy79
Case Problem:Advertising Strategy79
CHAPTER THREE Linear Programming:Formulation,Computer Solution,and Interpretation81
3.1 Computer Solution of Linear Programs81
Interpretation of Computer Output82
Simultaneous Changes85
Interpretation of Computer Output—A Second Example87
Cautionary Note on the Interpretation of Dual Prices89
3.2 More Than Two Decision Variables90
The Modified Par,Inc.,Problem90
The Bluegrass Farms Problem95
Formulation of the Bluegrass Farms Problem95
Computer Solution and Interpretation for the Bluegrass Farms Problem97
Guidelines for Model Formulation99
3.3 Modeling99
Management Science in Action:An Optimal Wood Procurement Policy100
The Electronic Communications Problem101
Formulation of the Electronic Communications Problem102
Computer Solution and Interpretation for the Electronic Communications Problem103
Management Science in Action:Using Linear Programming for Traffic Control106
Glossary107
Summary107
Problems108
case Problem:Product Mix121
Case Problem:Truck Leasing Strategy122
Appendix 3.2:Solving Linear Programs with LINDO/PC123
Appendix 3.1:Solving Linear Programs with The Management Scientist123
Appendix 3.3:Spreadsheet Solution of Linear Programs126
Management Science in Practice:Eastman Kodak130
CHAPTER FOUR Linear Programming Applications132
4.1 Marketing Applications132
Media Selection132
Marketing Research136
Portfolio Selection139
4.2 Financial Applications139
Financial Planning143
Management Science in Action:Using Linear Programming for Optimal Lease Structuring143
A Make-or-Buy Decision147
4.3 Production Management Applications147
Production Scheduling150
Management Science in Action:Libbey-Owens-Ford157
Work-Force Assignment157
4.4 Blending Problems161
Evaluating the Performance of Hospitals166
4.5 Data Envelopment Analvsis166
An Overview of the DEA Approach167
The DEA Linear Programming Model168
Summary of the DEA Approach172
Summary173
Problems174
Case Problem:Environmental Protection187
Case Problem:Investment Strategy189
Case Problem:Textile Mill Scheduling190
Appendix 4.1 Spreadsheet Solution of Linear Programs191
Management Science in Practice:Marathon Oil Company193
CHAPTET FIVE Linear Programming:The Simplex Method195
5.1 An Algebraic Overview of the Simplex Method195
Management Science in Action:Fleet Assignment at Delta Air Lines196
Algebraic Properties of the Simplex Method197
Determining a Basic Solution197
5.2 Tableau Form198
Basic Feasible Solutions198
5.3 Setting Up the Initial Simplex Tableau200
5.4 Improving the Solution203
5.5 Calculating the Next Tableau205
Interpreting the Results of an Iteration207
Moving toward a Better Solution208
Interpreting the Optimal Solution210
Summary of the Simplex Method211
5.6 Tableau Form:The General Case212
Greater-Than-or-Equal-to Constraints213
Equality Constraints217
Eliminating Negative Right-Hand-Side Values217
Summary of the Steps to Create Tableau Form218
5.7 Solving a Minimization Problem220
5.8 Special Cases222
Infeasibility222
Unboundedness223
Altemative Optimal Solutions224
Degeneracy226
Summary227
Problems229
Glossary229
Objective Function Coefficients239
6.1 Sensitivity Analysis with the Simplexrableau239
CHAPTER SIX Simplex-Based Sensitivity Analysis and Duality239
Right-Haod-Side Values244
Simultaneous Changes250
6.2 Duality251
Economic Interpretation of the Dual Variables254
Using the Dual to Identify the Primal Solution255
Findingthe Dual of Anv Primal Problem256
Glossary258
Summary258
Problems259
Management Science in Practice:Performance Analysis Corporation266
CHAPTER SEVEN Transportation,Assignment,and Transshipment Problems268
7.1 The Transportation Problem:The Network Model and a Linear Programming Formulation268
Problem Variations273
A General Linear Programming Model of the Transportation Problem274
7.2 The Assignment Problem:The Network Model and a Linear Programming Formulation276
Management Science in Action:Marine Corps Mobilization276
Problem Variations279
Multiple Assignments280
A General Linear Programming Model of the Assignment Problem280
7.3 The Transshipment Problem:The Nelwork Model and a Linear Programming Formulation281
Problem Variations286
A General Linear Programming Model of the Transshipment Problem287
7.4 A Production and Inventory Application288
7.5 The Transportation Simplex Method:A Special-Purpose Solution Procedure(Optional)291
Phase Ⅰ:Finding an Initial Feasible Solution292
Phase Ⅱ:Iterating to the Optimal Solution296
Summary of the Transportation Simplex Method305
Problem Variations306
7.6 The Assignment Problem:A Special-Purpose Solution Procedure(Optional)307
Finding the Minimum Number of Lines310
Problem Variations310
Summary313
Glossary314
Problems315
Case Problem:Assigning Umpire Crews330
Case Problem:Distribution System Design332
Management Science jn Practice:Procter Gamble334
CHAPTER EIGHT Integer Linear Programming335
Management Science in Action:Scheduling Employees at McDonald's Restaurant336
8.1 Types of Integer Linear Programming Models336
8.2 Graphicaland Computer Solution for an All-Integer Linear Program338
Graphical Solution Procedure338
Computer Solution341
Management Science in Action:Cutting Photographic Color Paper Rolls341
8.3 Applications342
Capital Budgeting342
Models Involving Fixed Costs344
Distribution System Design346
ABank Location Application350
8.4 Modeling Flexibility Provided by 0-1 Integer Variables354
Multiple-Choice and Mutually Exclusive Constraints355
Management Science in Action:Analyzing Price Quotations Under Business Volume Discounts355
k Out of n Alternatives Constraint356
Conditional and Corequisite Constraints356
Summary357
A Cautionary Note on Sensitivity Analysis357
Problems358
Glossary358
Case Problem:Textbook Publishing367
Case Problem:Production Scheduling with Changeover Costs369
Management Science in Practice:Ketron370
CHAPTER NINE Network Models372
9.1 The Shortest-Route Problem372
A Shortest-Route Algorithm373
A Minimal Spanning Tree Algorithm381
9.2 The Minimal Spanning Tree Problem381
9.3 The Maximal Flow Problem384
A Maximal Flow Algorithm385
Glossary390
Problems390
Summary390
Case Problem:Ambulance Routing397
Management Science in Practice:EDS399
CHAPTER TEN Project Scheduling:PERT/CPM401
10.1 Project Scheduling with Known Activity Times402
The Concepts of a Critical Path403
Determining the Critical Path404
Contributions of PERT/CPM409
Management Science in Action:Project Management on the PC410
Summary of the PERT/CPM Critical Path Procedure411
The Daugherty Porta-Vac Project412
10.2 Project Scheduling with Uncertain Activity Times412
Uncertain Activity Times413
The Critical Path415
Variability in Project Completion Time418
10.3 Considering Time-Cost Trade-Offs420
Crashing Activity Times421
A Linear Programming Model for Crashing Decisions423
Summary425
Glossary425
Problems426
Case Problem:Warehouse Expansion435
Management Science in Practice:Seasongood Mayer436
CHAPTER ELEVEN Inventory Models439
11.1 Economic Order Quantity(EOQ)Model 440
The How-Much-to-Order Decision443
The When-to-Order Decision445
Sensitivity Analysis in the EOQ Model446
The Manager's Use of the EOQ Model446
A Summary of the EOQ Model Assumptions447
How Has the EOQ Decision Model Helped?447
11.2 Economic Production Lot Size Model448
The Total Cost Model449
Finding the Economic Production Lot Size451
11.4 Quantity Discounts for the EOQ Model452
11.3 An Inventory Model with Planned Shortages452
11.5 A Single-Period lnventory Model with Probabilistic Demand458
The Johnson Shoe Company Problem459
The Kremer Chemical Company Problem462
11.6 An Order-Quantity,Reorder-Point Model with Probabilistic Demand464
The When-to-Order Decision466
The How-Much-to-Order Decision466
11.7 A Periodic-Review Model with Probabilistic Demand468
Management Science in Action:Information from a Netherlands Supplier Lowers Inventory Cost468
More Complex Periodic-Review Models471
Management Science in Action:Inventory Model Helps Hewlett-Packard's Product Design for Worldwide Markets471
11.8 Material Requirements Planning472
Dependent Demand and the MRP Concept473
Information System for MRP474
MRP Calculations476
11.9 The Just-in-Time Approach to Inventory Management478
Summary479
Glossary479
Problems481
Case Problem:A Make-or-Buy Analysis485
Appendix 11.1:Inventory Models with Spreadsheets489
Appendix 11.3 Development of the Optimal Lot Size(Q)Formula for the Production Lot Size Model492
Appendix 11.2 Development of the Optimal Order-Quantity(Q)Formula for the EOQ Model492
Appendix 11.4 Development of the Optimal Order-Quantity(Q)and Optimal Backorder(S)Formulas for the Planned Shortage Model493
Management Science in Practice:SupeRx.Inc.495
CHAPTER TWELVE Waiting Line Models497
12.1 The Structure of a Waiting Line System498
The Single-Channel Waiting Line498
The Distribution of Arrivals498
The Distribution of Service Times499
Queue Discipline500
Steady-State Operation500
The Operating Characteristics501
12.2 The Single-Channel Waiting Line Model with Poisson Arrivals and Exponential Service Times501
Operating Characteristics for the Burger Dome Problem502
The Manager's Use of Waiting Line Models503
Improving the Waiting Line Operation503
12.3 The Multiple-Channel Waiting Line Model with Poisson Arrivals and Exponential Service Times504
The Operatinig Characteristics505
Operating Characteristics for the Burger Dome Problem506
Management Science in Action:Hospital Staffing Based on a Multiple-Channel Waiting Line Model509
12.4 Some General Relationships for Waiting Line Models509
12.5 Economic Analysis of Waiting Lines511
12.6 Other Waiting Line Models513
Operating Characteristics for the M/G/l Model514
12.7 The Single-Channel Waiting Line Model with Poisson Arrivals and Arbitrary Service Times514
Constant Service Times515
12.8 A Multiple-Channel Model with Poisson Arrivals,Arbitrary Service Times,and No Waiting Line516
The Operating Characteristics for the M/G/K Model with Blocked Customers Cleared517
The Operating Characteristics for the M/M/l Model with a Finite Calling Population519
12.9 Waiting Line Models with Finite Calling Populations519
Summary522
Management Science in Action:Improving Fire Department Productivity522
Glossary523
Problems523
Case Problem:Airline Reservations530
Appendix 12.1:Waiting Line Models with Spreadsheets531
Management Science in Practice:CITIBANK533
CHAPER THIRETTEN Simulation535
13.1 Using Simulation for Risk Analysis536
The PortaCom Project536
The PortaCom Simulation Model537
Random Numbers and Simulating Values of Random Variables539
Using the Simulation Model541
Risk Analysis Conclusions542
Simulation Results542
13.2 An Inventory Simulation Model543
Some Simulation Terminology543
13.3 A Waiting Line Simulation Model546
The Hammondsport Savings and Loan Waiting Line546
Customer Arrival Times546
Customer Service Times547
The Simulation Model548
Simulation Results551
Management Science in Action:Red Cross Uses Simulation to Improve Bloodmobile Services553
Selecting a Simulation Language554
13.4 Other lssues554
Verification and Validation555
Keeping Track of Time556
Advantages and Disadvantages556
Management Science in Action:Simulation at Mexico's Vilpac Truck Company557
Summary557
Glossary558
Problems558
Case Problem:County Beverage Drive-Thru567
Case Problem:Machine Repair568
Appendix 13.1 Simulation with Spreadsheets569
Management Science in Practice:The Upjohn Company573
CHAPTER FOURTEEN Decision Analysis575
Payoff Tables576
14.1 Structuring the Decision Problem576
Decision Trees577
14.2 Decision Making Without Probabilities578
Optimistic Approach579
Conservative Approach579
Minimax Regret Approach580
14.3 Decision Making with Probabilities581
14.4 Sensitivity Analvsis584
Management Science in Action:Decision Analysis and the Selection of Home Mortgages584
14.5 Expected Value of Perfect Information587
14.6 Decision Analvsis with Sample Information589
14.7 Developing a Decision Strategy591
Computing Branch Probabilities592
An Optimal Decision Strategy594
Management Science in Action:Decision Analysis and Drug Testing for Student Athletes596
14.8 Expected Value of Sample Information597
Efficiency of Sample Information598
The Meaning of Utility599
14.9 Utility and Decision Making599
Developing Utilities for Payoffs600
The Expected Utility Approach603
Glossary605
Summary605
Problems606
Case Problem:Property Purchase Strategy622
Appendix 14.1:Decision Analysis and Spreadsheets623
Management Science in Practice:Ohio Edison Company627
CHAPTET FIFTEEN Multicriteria Decision Problems630
15.1 Goal Programming:Formulation and Graphical Solution631
Developing the Constraints and the Goal Equations632
Developing an Objective Function with Preemptive Priorities633
The Graphical Solution Procedure634
The Goal Programming Model638
15.2 Goal Programming:Solving More Complex Problems639
The Suncoast Office Supplies Problem639
Formulating the Goal Equations640
Formulating the Objective Function641
Computer Solution643
15.3 The Analytic Hierarchy Process646
Management Science in Action:Using AHP and Goal Programming to Plan Facility Locations647
Developing the Hierarchy648
15.4 Establishing Priorities Using AHP648
The Pairwise Comparison Matrix649
Pairwise Comparisons649
Procedure for Synthesizing Judgments650
Synthesis650
Consistency651
Estimating the Consistency Ratio652
Other Pairwise Comparisons for the Car-Selection Problem653
15.5 Using AHP to Develop an Overall Priority Ranking655
15.6 Using Expert Choice to Implement AHP656
Summary659
Glossary660
Problems661
Case Problem:Production Scheduling668
CHAPTER XIXTEEN Forecasting669
16.1 The Components of a Time Series670
Trend Component671
Cyclical Component672
Seasonal Component672
Irregular Component673
16.2 Smoothing Methods673
Moving Averages673
Weighted Moving Averages676
Exponential Smoothing676
16.3 Trend Projection681
16.4 Trend and Seasonal Components684
The Multiplicative Model685
Calculating the Seasonal Indexes685
Deseasonalizing the Time Series689
Using the Deseasonalized Time Series to Identify Trend690
Seasonal Adjustments692
Models Based on Monthy Data692
Cyclical Component692
16.5 Forecasting Using Regression Models693
Management Science in Action:Spare Parts Forecasting at American Airlines693
Using Regression Analysis When Time Series Data Are Not Available694
Using Regression Analysis with Time Series Data698
Delphi Method700
16.6 Qualitative Approaches to Forecasting700
Scenario Writing701
Expert Judgment701
Management Science in Action:The Business Week Industry Outlook 70lIntuitive Approaches702
Summary702
Glossary702
Problems703
Case Problem:Forecasting Sales712
Case Problem:Forecasting Lost Sales713
Appendix 16.1 Forecasting with Spreadsheets714
Management Science in Practice:The Cincinnati Gas Electric Company716
CHAPTER SEVENTEEN Markov Processes718
17.1 Market Share Analysis718
17.2 Accounts Receivable Analysis726
The Fundamental Matrix and Associated Calculations727
Establishing the Allowance for Doubtful Accounts729
Problems731
Glossary731
Summary731
Management Science in Practice:U.S.General Accounting Office735
18.1 A Shortest-Route Problem737
CHAPTER ELGHTEEN Dynamic Programming737
18.2 Dynamic Programming Notation742
18.3 The Knapsack Problem745
18.4 A Production and Inventory Control Problem751
Summary755
Glossary755
Problems756
Management Science in Practice:The U.S.Environmental Protection Agency762